See what your fellow, Ambitious B2b Marketers have to say about Oceanfrogs
OceanFrogs has helped understand the total addressable Market for us in Pharmaceuticals, USA.
Manish Sharma CEO, Tika Mobile 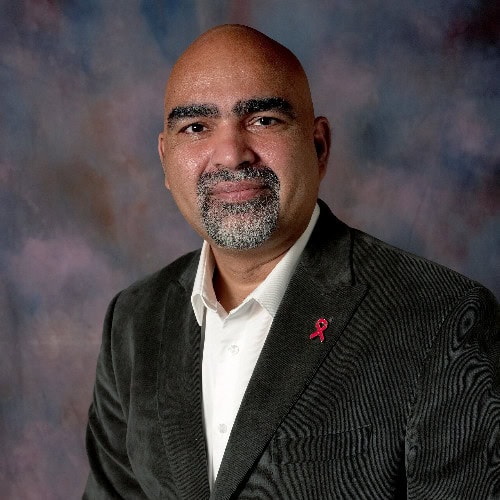
"The perfect platform for market intelligence"
What do you like best?
The accuracy of the data on the platform, The integrations possible for seamless marketing execution , A team that drives to deliver and hyper focusses on customer satisfaction
Aathira NairProduct Marketing Manager, MontyCloud What do you like best?
The accuracy of the data on the platform, The integrations possible for seamless marketing execution , A team that drives to deliver and hyper focusses on customer satisfaction
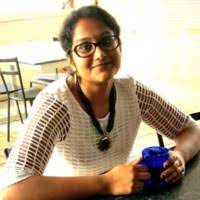
Build Channel Partners Ecosystem with OceanFrogs Sales Intelligence. The team is super friendly and helps you at each step of the way.
Manisha Singh Head of Marketing, 42Gears 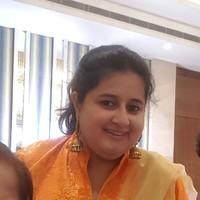
Actionable Intelligence that could be used by demand generation or Inside Sales team.
Ajit BelaniHead of Marketing, Eka Software 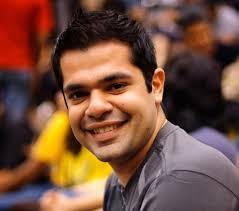
Team is very knowledgeable, made of data scientists, customer-focused, able to provide a personalized onboarding experience.
Shobhit MathurChief Business Officer 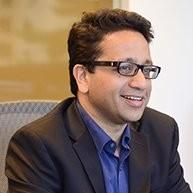
Oceanfrogs is a treasure trove of information. In the year of working with them, we got just one data record incorrect from the thousands that we mined. They were prompt and fixed that within hours. I love the speed and high degree of orientation to quality. The intent signals are pure gold.
Sudarsan RaviFounder and CEO, RippleHire 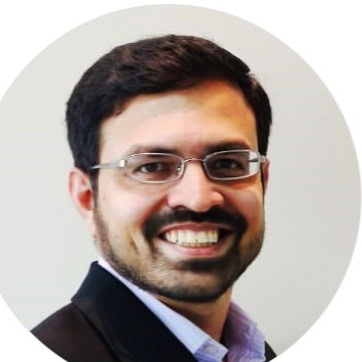
Previous
Next